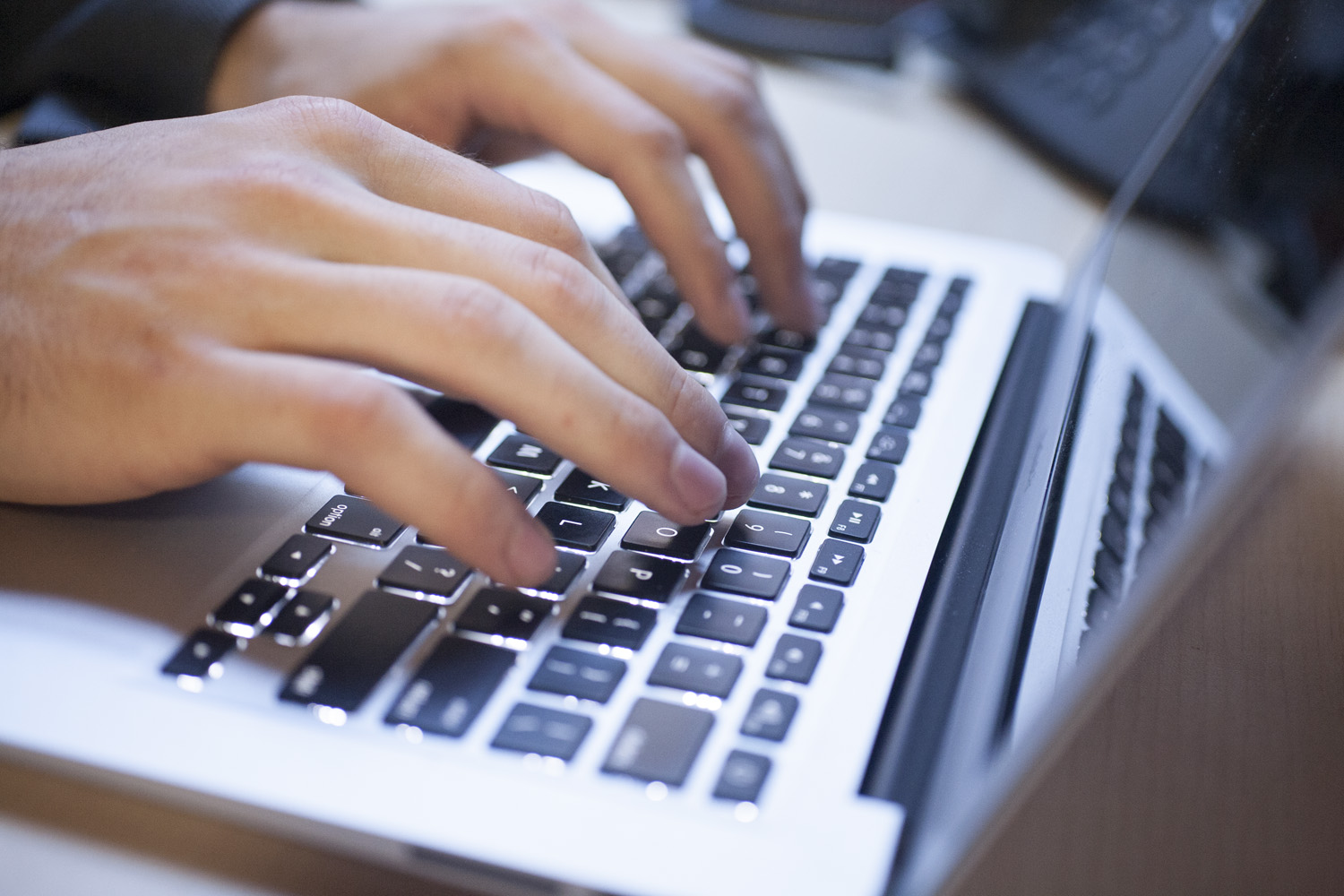
Customer service and moving from the enterprise to the flexiprise – Interview with Keith Leimbach
August 23, 2017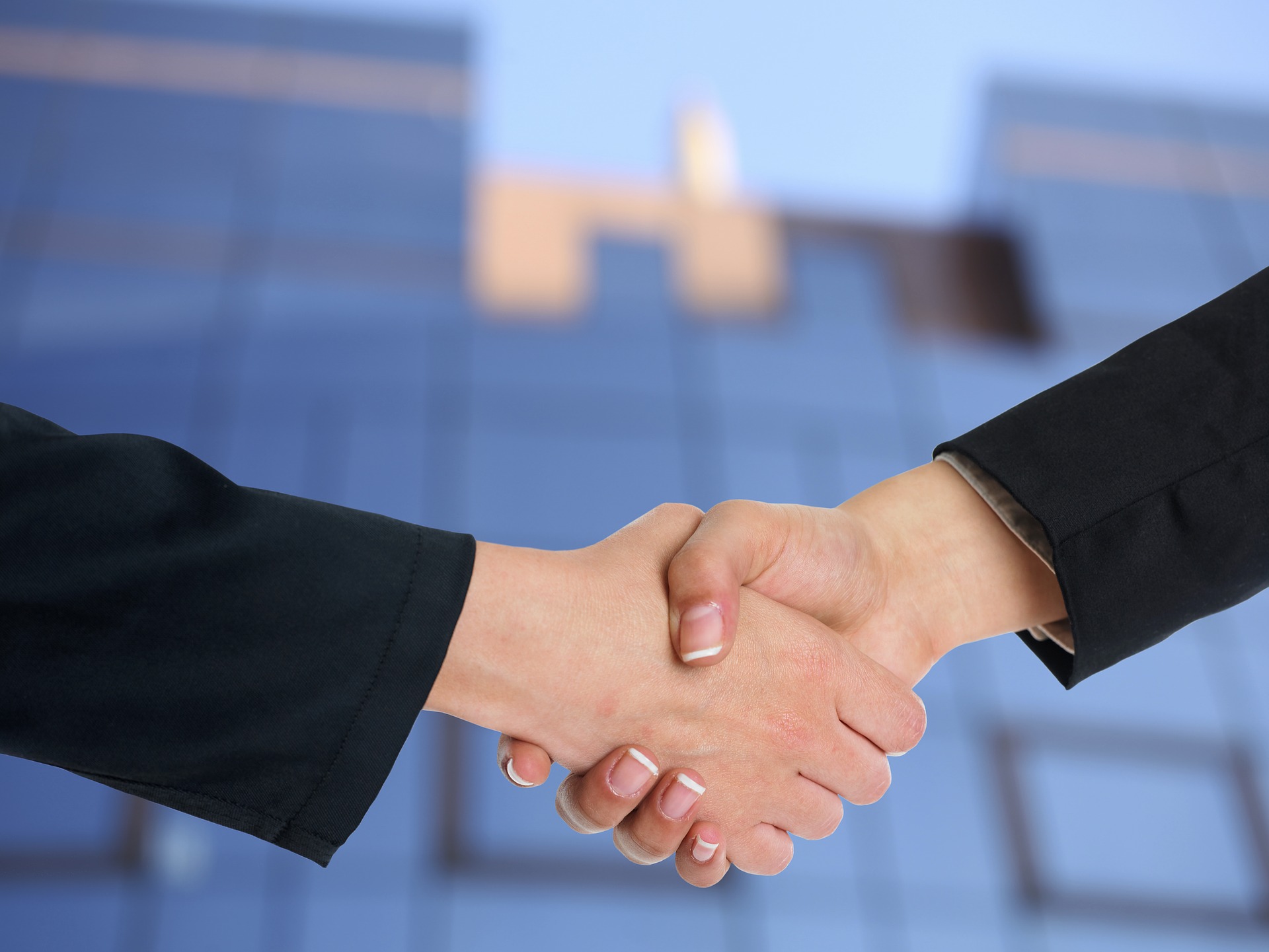
Marrying self organising teams and customer obsession – Interview with Andrew Lawson
September 4, 2017In recent days we have seen an escalation in the war of words between Elon Musk and Mark Zuckerberg surrounding the dangers of artificial intelligence (AI).
Musk worries that if unregulated AI will grow and grow in influence and could ultimately pose an existential threat to humanity. As a result, he is advocating that governments need to start regulating the technology.
Zuckerberg, on the other hand, disagrees on the need for more regulation and is more sanguine about the prospects of AI.
Now, this is a macro level argument about the prospects and nature of AI. And, it is one that is set to rumble on and on.
But, there is a more micro level challenge facing firms that are using AI technology right now.
This is particularly relevant for organizations that are using AI to enhancing their customer experience by making it more personalized, making their service more proactive or using algorithms and data sets to predict the most likely outcome of a particular situation, the next best offer or next best action for a customer.
The challenge was articulated by Dr. Rob Walker, Vice President, Decision Management and Analytics at Pegasystems, during a keynote speech at Pegaworld that took place in the early part of June in Las Vegas.
In his keynote, Rob explained that there are two types of AI. The first is Transparent AI, a system built around a machine learning algorithm that can explain how it works and can be audited.
The second is Opaque AI, a system, again built around a machine learning algorithm, that is more ‘black box’ in nature and one that cannot intrinsically explain itself and cannot be audited.
[Note: You can watch Rob’s keynote here and here is a link to a follow up discussion that I conducted with him for my podcast.]
Now, Opaque AI systems tend to be more powerful than Transparent AI systems, given that requiring a system to have to ‘explain’ itself and to be audit-able can tend to act as a ‘brake’ or restraint on its effectiveness and analytical ‘horse-power’. And, given their power they are likely to prove increasingly popular amongst organizations that are searching for tools and technology to help them differentiate themselves and deliver better business and customer outcomes.
But, Opaque AI comes with its own set of risks. It may be more powerful than a transparent system but, because of its nature, we are also limited in understanding what sort of attitudes it will develop and what outputs it might generate.
Remember Microsoft’s racist AI chatbot? Or, How Target Figured Out A Teen Girl Was Pregnant Before Her Father Did? We don’t want a repeat of those incidences, right?
As such, companies need to make conscious choices about what type of AI technology they want to use (Transparent or Opaque), how they use it and when to use it.
Dr Nicola Millard, Head of Customer Insight & Futures within BT’s Global Services Innovation Team brings this choice to life in an upcoming whitepaper (Botman vs. Superagent) when she writes:
“The debate extends to robo-advice in the financial services industry, and medical diagnosis. If a person is given a personalized recommendation based on the output of a machine learning algorithm, how is that advice regulated if the learning algorithm can’t show us how it came to that particular conclusion? ”
In the face of such challenges, Rob Walker suggests that firms are likely choose to deploy Transparent AI systems in areas that are subject to regulation, compliance and risk management issues.
However, in others they are likely to adopt the use of Opaque AI.
But, given the ‘black box’ nature of Opaque AI, they will need to be accompanied by testing systems that establish the attitudes and biases that the system is developing. In addition, its outputs will also need to be subject to ‘ethical’ and quality sign off mechanisms in order to make sure that they comply with existing laws, regulation, brand policies, customer promises and company procedures etc.
Now, these mechanisms do not need to be markedly different from other established governance and quality policies and procedures that normally exist but they will need to be updated to take into account of the impact and risks of using this type of AI and then built into existing operations.
Nicola Millard describes the situation very well when she says:
“As with any system, AI is very much a case of “garbage in, garbage out”. If we want AI that produces “good” answers, we need to feed it “good” data. We need to be responsible parents, teach it, supervise it, give it a healthy data diet, and work alongside it, rather than leaving it to its own devices”.
However, right now, it is not clear that these type of practices are fully developed or widespread.
But, they should be.
To not have them in place risks opening up your organization, your customer experience and your customers to some potentially damaging and unintended consequences.
This post was originally published on Forbes.com.